Credit card requirements and minimum eligibility criteria to fulfill
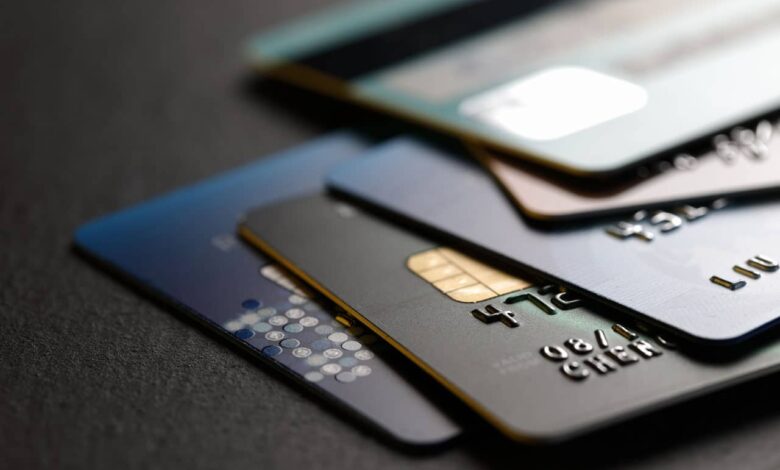
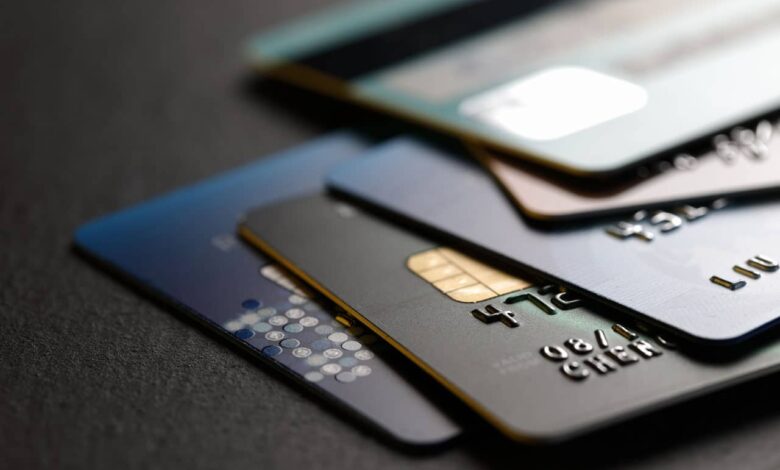
Credit cards, while becoming increasingly popular payment instruments, are also a handy way of accessing funds during emergencies. A report stated that the number of credit card users in India in 2019 touched 52 million, which only keeps on increasing for good.
The data also shows an overall increase in first-time credit card applicants sending the request for a credit card online. Yet, a majority of individuals lack the information on the right process to apply for a credit card, whether online or offline.
A prospective applicant must read the terms of eligibility and credit card interest rates beforehand to avoid accruing unnecessary debts in the future. Such information will also aid a cardholder in balancing the difference between expenditure and saving.
What are the eligibility criteria to apply for a credit card?
A credit card application needs to qualify certain criteria to receive approval for the payment card without any hindrances. The financial institutions mandate these criteria which check a borrower’s creditworthiness and reliability while ensuring whether he/she will be able to pay the bills in time without straining the monthly finances.
Here is a list of things to know before applying for a credit card along with the standard eligibility criteria required by the card provider. Take a look.
CIBIL score
An applicant holding a credit score above 750 is considered to carry a high creditworthiness. In effect, there is a greater chance of quick approval that too at a lower interest rate.
Similarly, a low credit score leads to a higher credit card interest rates applicability or refusal of the application altogether. An individual, therefore, needs to maintain an impressive credit history and a high score to avail of the credit card without any hassle.
Age limit
As a standard requirement, an applicant needs to be at least 25 years old to avail of the card. The minimum and maximum age requirements vary according to a financial institution’s policy, whereby most of them permit upto 65 years as the age limit to apply for a credit card.
Nationality
Many NBFCs cater to the Indian market that includes both domestic and foreign applicants. Ideally, financial institutions require individuals to be Indian residents to apply for credit cards and other loans.
It is suggested to check the actual eligibility criteria with the specific lenders before applying for a credit card. It will help an individual to collect the necessary documents beforehand.
Stable income profile
An applicant’s income plays a significant bearing in credit card approval. Salaried employees with stable earnings from reputed organizations are usually preferred by card issuers for a higher credit limit. Income stability is, however, also a lookout in the case of self-employed applicants, with businesses operating for more than a few years bearing an increased capacity to repay their credit card dues.
Authentic documents
While not among the eligibility criteria, appropriate documentation is an essential requirement, whereby applicants need to complete the paperwork to successfully apply for a credit card.
Some of the standard documents every financial institution requires are-
1. Aadhaar card.
2. Driving license.
3. Passport.
4. Voter ID card.
5. Salary slip.
6. PAN card.
7. Nationality proof.
8. Bank account statement.
Apart from the ones mentioned, an applicant might have to submit additional documents as asked for by the financial institution. Moreover, the documents can differ for salaried, non-salaried, and self-employed individuals.
No default in the credit profile
A financial institution also checks an applicant’s profile before approving the credit card. A default or an individual with accrued debts will stand a reduced chance of availing approval for the card. Therefore, maintaining a good profile in terms of credit is mandatory to choose the right credit card.
Apart from these factors, cards like the Bajaj Finserv RBL Bank SuperCard also come with multiple added benefits to enhance the cardholder’s experience.
Further, the card’s issuer also provides pre-approved offers on financial products like credit cards, business loans, personal loan, etc. to simplify credit processing within minimum time. You can check your pre-approved offer by entering a name and contact number.
While credit cards enhance a users’ spending ability, using them relentlessly can accrue unwanted debts and unnecessary strain on the finances. Therefore, one must apply for the card when he/she is confident about the repayment capability and eligibility.